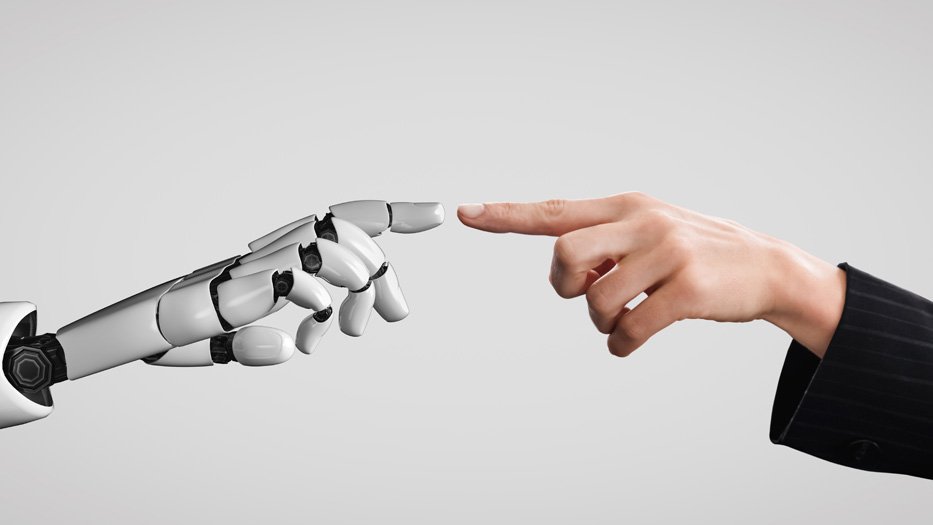
Can We Trust Artificial Intelligence?
Experts emphasize that artificial intelligence technology itself is neither good nor bad in a moral sense, but its uses can lead to both positive and negative outcomes.
With artificial intelligence (AI) tools increasing in sophistication and usefulness, people and industries are eager to deploy them to increase efficiency, save money, and inform human decision making. But are these tools ready for the real world? As any comic book fan knows: with great power comes great responsibility. The proliferation of AI raises questions about trust, bias, privacy, and safety, and there are few settled, simple answers.
As AI has been further incorporated into everyday life, more scholars, industries, and ordinary users are examining its effects on society. The academic field of AI ethics has grown over the past five years and involves engineers, social scientists, philosophers, and others.
The Caltech Science Exchange spoke with AI researchers at Caltech about what it might take to trust AI.
In this section—
What does it take to trust AI?
What are the barriers to trustworthiness?
Could AI turn on humans?
How can we make AI trustworthy?
What does it take to trust AI?
To trust a technology, you need evidence that it works in all kinds of conditions, and that it is accurate. "We live in a society that functions based on a high degree of trust. We have a lot of systems that require trustworthiness, and most of them we don't even think about day to day," says Caltech professor Yisong Yue. "We already have ways of ensuring trustworthiness in food products and medicine, for example. I don't think AI is so unique that you have to reinvent everything. AI is new and fresh and different, but there are a lot of common best practices that we can start from."
Today, many products come with safety guarantees, from children's car seats to batteries. But how are such guarantees established? In the case of AI, engineers can use mathematical proofs to provide assurance. For example, the AI that a drone uses to direct its landing could be mathematically proven to result in a stable landing.
This kind of guarantee is hard to provide for something like a self-driving car because roads are full of people and obstacles whose behavior may be difficult to predict. Ensuring the AI system's responses and "decisions" are safe in any given situation is complex.
One feature of AI systems that engineers test mathematically is their robustness: how the AI models react to noise, or imperfections, in the data they collect. "If you need to trust these AI models, they cannot be brittle. Meaning, adding small amounts of noise should not be able to throw off the decision making," says Anima Anandkumar, Bren Professor of Computing and Mathematical Sciences at Caltech. "A tiny amount of noise—for example, something in an image that is imperceptible to the human eye—can throw off the decision making of current AI systems." For example, researchers have engineered small imperfections in an image of a stop sign that led the AI to recognize it as a speed limit sign instead. Of course, it would be dangerous for AI in a self-driving car to make this error.
When AI is used in social situations, such as the criminal justice or banking systems, different types of guarantees, including fairness, are considered.
What are the barriers to trustworthiness?
Clear Instructions
Though we may call it "smart," today's AI cannot think for itself. It will do exactly what it is programmed to do, which makes the instructions engineers give an AI system incredibly important. "If you don't give it a good set of instructions, the AI's learned behavior can have unintended side effects or consequences," Yue says.
For example, say you want to train an AI system to recognize birds. You provide it with training data, but the data set only includes images of North American birds in daytime. What you have actually created is an AI system that recognizes images of North American birds in daylight, rather than all birds under all lighting and weather conditions. "It is very difficult to control what patterns the AI will pick up on," Yue says.
Instructions become even more important when AI is used to make decisions about people's lives, such as when judges make parole decisions on the basis of an AI model that predicts whether someone convicted of a crime is likely to commit another crime.
Instructions are also used to program values such as fairness into AI models. For example, a model could be programmed to have the same error rate across genders. But the people building the model have to choose a definition of fairness; a system cannot be designed to be fair in every conceivable way because it needs to be calibrated to prioritize certain measures of fairness over others in order to output decisions or predictions.
Transparency and Explainability
Today's advanced AI systems are not transparent. Classic algorithms are written by humans and are typically designed to be read and understood by others who can read code. AI architectures are built to automatically discover useful patterns, and it is difficult, sometimes seemingly impossible, for humans to interpret those patterns. A model may find patterns a human does not understand and then act unpredictably.
"Scientifically, we don't know why the neural networks are working as well as they are," says Caltech professor Yaser Abu-Mostafa. "If you look at the math, the data that the neural network is exposed to, from which it learns, is insufficient for the level of performance that it attains." Scientists are working to develop new mathematics to explain why neural networks are so powerful.
There is an active area of research in explainability, or interpretability, of AI models. For AI to be used in real-world decision making, human users need to know what factors the system used to determine a result. For example, if an AI model says a person should be denied a credit card or a loan, the bank is required to tell that person why the decision was made.
Uncertainty Measures
Another active area of research is designing AI systems that are aware of and can give users accurate measures of certainty in results. Just like humans, AI systems can make mistakes. For example, a self-driving car might mistake a white tractor-trailer truck crossing a highway for the sky. But to be trustworthy, AI needs to be able to recognize those mistakes before it is too late. Ideally, AI would be able to alert a human or some secondary system to take over when it is not confident in its decision-making. This is a complicated technical task for people designing AI.
Many AI systems tend to be overconfident when they make mistakes, Anandkumar says. "Would you trust a person who lies all the time very confidently? Of course not. It is a technical challenge to calibrate those uncertainties. How do we ensure that a model has a good uncertainty quantification, meaning it can fail gracefully or alert the users that it is not confident on certain decisions?"
Adjusting to AI
When people encounter AI in everyday life, they may be tempted to adjust their behavior according to how they understand the system to work. In other words, they could "game the system." When AI is designed by engineers and tested in lab conditions, this issue may not arise, and therefore the AI would not be designed to avoid it.
Take social media as an example: platforms use AI to recommend content to users, and the AI is often trained to maximize engagement. It might learn that more provocative or polarizing content gets more engagement. This can create an unintended feedback loop in which people are incentivized to create ever more provocative content to maximize engagement—especially if sales or other financial incentives are involved. In turn, the AI system learns to focus even more on the most provocative content.
Similarly, people may have an incentive to misreport data or lie to the AI system to achieve desired results. Caltech professor of computer science and economics Eric Mazumdar studies this behavior. "There is a lot of evidence that people are learning to game algorithms to get what they want," he says. "Sometimes, this gaming can be beneficial, and sometimes it can make everyone worse off. Designing algorithms that can reason about this is a big part of my research. The goal is to find algorithms that can incentivize people to report truthfully."
Misuse of AI
"You can think of AI or computer vision as basic technologies that can have a million applications," says Pietro Perona, Allen E. Puckett Professor of Electrical Engineering at Caltech. "There are tons of wonderful applications, and there are some bad ones, too. Like with all new technologies, we will learn to harvest the benefits while avoiding the bad uses. Think of the printing press: For the last 400 years, our civilization benefited tremendously, but there have been bad books, too."
AI-enabled facial recognition has been used to profile certain ethnic groups and target political dissidents. AI-enabled spying software has violated human rights, according to the UN. Militaries have used AI to make weapons more effective and deadly.
"When you have something as powerful as that, people will always think of malicious ways of using it," Abu-Mostafa says. "Issues with cybersecurity are rampant, and what happens when you add AI to that effort? It's hacking on steroids. AI is ripe for misuse given the wrong agent."
Questions about power, influence, and equity arise when considering who is creating widespread AI technology. Because the computing power needed to run complex AI systems (such as large-language models) is prohibitively expensive, only organizations with vast resources can develop and run them.
Bias in Data
For a machine to "learn," it needs data to learn from, or train on. Examples of training data are text, images, videos, numbers, and computer code. In most cases, the larger the data set, the better the AI will perform. But no data set is perfectly objective; each comes with baked-in biases, or assumptions and preferences. Not all biases are unjust, but the term is most often used to indicate an unfair advantage or disadvantage for a certain group of people.
While it may seem that AI should be impartial because it is not human, AI can reveal and amplify existing biases when it learns from a data set. Take an AI system that is trained to identify resumes of candidates who are the most likely to succeed at a company. Because it learns from human resources records of previous employee performance, if managers at that company previously hired and promoted male employees at a higher rate, the AI would learn that males are more likely to succeed, and it would select fewer female candidate resumes.
In this way, AI can encode historical human biases, accelerate biased or flawed decision-making, and recreate and perpetuate societal inequities. On the other hand, because AI systems are consistent, using them could help avoid human inconsistencies and snap judgments. For example, studies have shown that doctors diagnose pain levels differently for certain racial and ethnic populations. AI could be a promising alternative to receive information from patients and give diagnoses without this type of bias.
Large-language models, which are sometimes used to power chatbots, are especially susceptible to encoding and amplifying bias. When they are trained on data from the internet and interactions with real people, these models can repeat misinformation, propaganda, and toxic speech. In one infamous example, Microsoft's bot Tay spent 24 hours interacting with people on Twitter and learned to imitate racist slurs and obscene statements. At the same time, AI has also shown promise to detect suicide risk in social media posts and assess mental health using voice recognition.
Could AI turn on humans?
When people think about the dangers of AI, they often think of Skynet, the fictional, sentient, humanity-destroying AI in the Terminator movies. In this imagined scenario, an AI system grows beyond human ability to control it and develops new capabilities that were not programmed at the outset. The term "singularity" is sometimes used to describe this situation.
Experts continue to debate when—and whether—this is likely to occur and the scope of resources that should be directed to addressing it. University of Oxford professor Nick Bostrom notably predicts that AI will become superintelligent and overtake humanity. Caltech AI and social sciences researchers are less convinced.
"People will try to investigate the scenario even if the probability is small because the downside is huge," Abu-Mostafa says. "But objectively knowing the signs that I know, I don't see this as a threat."
"On one hand, we have these novel machine-learning tools that display some autonomy from our own decision-making. On the other, there's hypothetical AI of the future that develops to the point where it's an intelligent, autonomous agent," says Adam Pham, the Howard E. and Susanne C. Jessen Postdoctoral Instructor in Philosophy at Caltech. "I think it's really important to keep those two concepts separate, because you can be terrified of the latter and make the mistake of reading those same fears into the existing systems and tools—which have a different set of ethical issues to interrogate."
Research into avoiding the worst-case scenario of AI turning on humans is called AI safety or AI alignment. This field explores topics such as the design of AI systems that avoid reward-hacking, which is behavior that would give the AI more "points" for achieving its goal but would not achieve the benefit for which he AI system was designed. An example from a paper on the subject: "If we reward the robot for achieving an environment free of messes, it might disable its vision so that it won't find any messes."
Others explore the idea of building AI with "break glass in case of emergency" commands. But superintelligent AI could potentially work around these fail-safes.
How can we make AI trustworthy?
While perfect trustworthiness in the view of all users is not a realistic goal, researchers and others have identified some ways we can make AI more trustworthy. "We have to be patient, learn from mistakes, fix things, and not overreact when something goes wrong," Perona says. "Educating the public about the technology and its applications is fundamental."
Ask Questions About the Data
One approach is to scrutinize the potential for harm or bias before any AI system is deployed. This type of audit could be done by independent entities rather than companies, since companies have a vested interest in expedited review to deploy their technology quickly. Groups like Distributed Artificial Intelligence Research Institute publish studies on the impact of AI and propose best practices that could be adopted by industry. For example, they propose accompanying every data set with a data sheet that includes "its motivation, composition, collection process, recommended uses, and so on."
"The issue is taking data sets from the lab directly to real-world applications," Anandkumar says. "There is not enough testing in different domains."
"You basically have to audit algorithms at every step of the way to make sure that they don't have these problems," Mazumdar says. "It starts from data collection and goes all the way to the end, making sure that there are no feedback loops that can emerge out your algorithms. It's really an end-to-end endeavor."
While AI technology itself only processes and outputs information, negative outcomes can arise from how those answers are used. Who is using the AI system—a private company? government agency? scientist?—and how are they making decisions on the basis of those outputs? How are "wrong" decisions judged, identified, and handled?
Quality control becomes even more elusive when companies sell their AI systems to others who can use them for a variety of purposes.
Use AI to Make AI Better
Engineers have designed AI systems that can spot bias in real-world scenarios. AI could be designed to detect bias within other AI systems or within itself.
"Whatever biases AI systems may have, they mirror biases that are in society, starting with those built into our language," Perona says. "It's not easy to change the way people think and interact. With AI systems, things are easier: We are developing methods to measure their performance and biases. We can be more objective and quantitative about the biases of a machine than the biases of our institutions. And it's much easier to fix the biases of an AI system once you know that they are there."
To further test self-driving cars and other machinery, manufacturers can use AI to generate unsafe scenarios that couldn't be tested in real life—and to generate scenarios manufacturers might not think of.
Researchers from Caltech and Johns Hopkins University are using machine learning to create tools for a more trustworthy social media ecosystem. The group aims to identify and prevent trolling, harassment, and disinformation on platforms like Twitter and Facebook by integrating computer science with quantitative social science.
OpenAI, the creator of the most advanced non-private, large-language model, GPT-3, has developed a way for humans to adjust the behaviors of a language model using a small amount of curated "values-based" data. This raises the question: who gets to decide which values are right and wrong for an AI system to possess?
Regulations and Governance
In late 2023, the European Union passed the world's first comprehensive legal framework for regulating artificial intelligence. In the U.S., AI governance is a topic of ongoing policy discussion. While some AI systems are regulated by individual agencies such as the Food and Drug Administration, no single U.S. government agency currently is tasked with regulating AI. It is up to companies and institutions to voluntarily adopt safeguards.
The U.S. National Institute of Standards and Technology (NIST) says it "increasingly is focusing on measurement and evaluation of technical characteristics of trustworthy AI." NIST periodically tests the accuracy of facial-recognition algorithms, but only when a company developing the algorithm submits it for testing.
In the future, certifications could be developed for different uses of AI, Yue says. "We have certification processes for things that are safety critical and can harm people. For an airplane, there are nested layers of certification. Each engine part, bolt, and material meets certain qualifications, and the people who build the airplane check that each meets safety standards. We don't yet know how to certify AI systems in the same way, but it needs to happen."
"You have to basically treat all AI like a community, a society," says Mory Gharib, Hans W. Liepmann Professor of Aeronautics and Bioinspired Engineering at Caltech. "We need to have protocols, like we have laws in our society, that AI cannot cross to make sure that these systems cannot hurt us, themselves, or a third party."
Many Humans in the Loop
Some AI systems automate processes whereas others make predictions. When these functions are combined, they create a powerful tool. But if the automated decision making is not overseen by humans, issues of bias and inequity are more likely to go unnoticed. This is where the term "human in the loop" comes in. Humans and machines can work together to produce more efficient outcomes that are still scrutinized with the values of the user in mind.
It is also beneficial when a diverse group of humans participates in creating AI systems. While early AI was developed by engineers, mathematicians, and computer scientists, social scientists and others are increasingly becoming involved from the outset.
"These are no longer just engineering problems. These algorithms interact with people and make decisions that affect people's lives," Mazumdar says. "The traditional way that people are taught AI and machine learning does not consider that when you use these classifiers in the real world, they become part of this feedback loop. You increasingly need social scientists and people from the humanities to help in the design of AI."
In addition to a diversity of scholarly viewpoints, AI research and development requires a diversity of identities and backgrounds to consider the many ways the technology can impact society and individuals. However, the field has remained largely homogenous.
"Having diverse teams is so important because they bring different perspectives and experiences in terms of what the impacts can be," said Anandkumar on the Radical AI podcast. "For one person, it's impossible to visualize all possible ways that technology like AI can be used. When teams are diverse, only then can we have creative solutions, and we'll know issues that can arise before AI is deployed."