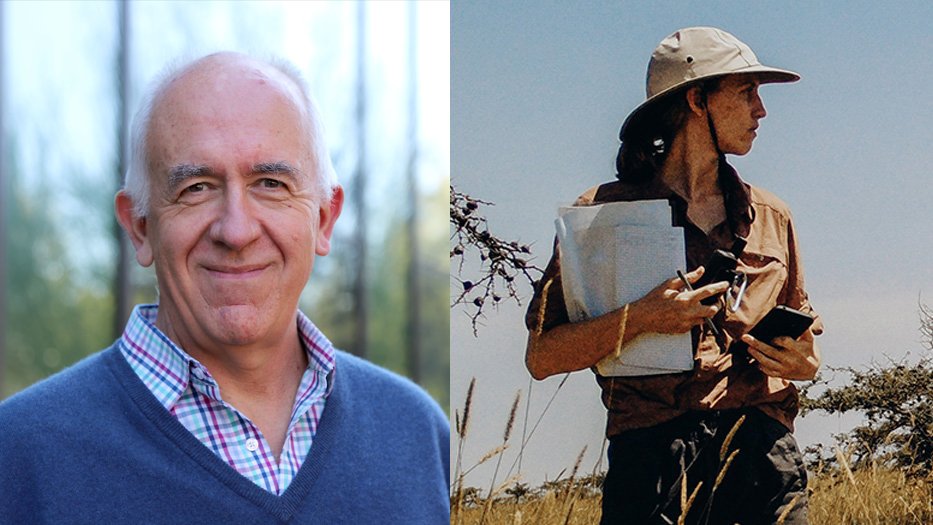
Ask a Caltech Expert: Pietro Perona and Sara Beery on AI for Conservation
How Do Researchers Use AI for Biodiversity?
Pietro Perona
Allen E. Puckett Professor of Electrical Engineering
By some estimates (e.g., a 2019 UN report), we are quickly losing species, and we strongly suspect that human activity is the main culprit. In order to know what's going on and, crucially, what to do about it, ecologists and field biologists need data—about species distributions, how ecosystems work, human impact, and the effects of climate change. The data that they have now are not sufficient. We need to cover more of the planet, observe more species. We need data that are more accurate. Here is where AI can help because it is simply not possible to keep collecting and analyzing data by hand. AI can help scale up from a few chosen spots to the whole planet, process the data faster, and handle data from a more diverse suite of sensors.
One of the domains in which we are working is monitoring species distribution through measurements and modeling. An example is salmon fishery management: the state of Alaska needs to know how many salmon make it all the way up the rivers each year to spawn, so that we have a sufficient number of reproducing pairs and the fishery is sustainable. In this case, we have ultrasound sensors in rivers, where we can obtain 24/7 video of fish migrating upstream. We are developing algorithms that can count the fish and classify their species automatically. Right now, it's all done by hand! Measurements can also be obtained by dropping microphones from a plane into a forest to pick up signals that identify species by the sounds that they make, setting out cameras that photograph their movement, or by crowdsourcing from photographs that people take in nature.
In terms of measuring species density, we can go one step further and track individuals of a species to learn about their range, where they travel, and their habits. There are ad hoc techniques for identifying different species. For whales, people look at scratches on the tail fins; for zebras and leopards, people look at specific spots; for lions, the coat. Nobody knows yet if we can reliably identify animals that don't have specific or special markings—for example, cougars—but that's certainly something that we are trying to do. We have a project called Visipedia that can identify whether the species is expanding or not to give us an idea of biodiversity.
Something interesting we are exploring is exploiting informal observations that are made by regular people and citizen scientists when they are out in nature. The iNaturalist app, which we have developed in collaboration with the California Academy of Sciences, is collecting millions of observations each year, and we are learning how to make sense of those data.
AI can also help us analyze satellite images of Earth. We can see the distribution of certain species of plants or trees and account for their levels of stress. For example, in a drought, we can count individual trees in dry areas and see if they're receding or if they're repopulating. We can also monitor human activity and estimate its impact on the environment. For example, we can train AI systems to automatically detect illegal mines from satellite images.
Sara Beery
Graduate student in computing and mathematical sciences
Computer vision gives scientists, policymakers, and conservationists the data they need to make close to real-time decisions about where to allocate resources. One example here in Los Angeles is the use of GPS collars for mountain lions. Camera traps have been placed at underpasses under the 101 freeway to figure out where the mountain lions are crossing and verify that they're crossing in a safe way. This information is being used to make the case for a new animal overpass over the 101.
There is an amazing diversity of ways that computer vision is being used for conservation, engaging with many different types of data and many different stakeholder needs. For that reason, it is important for computer scientists like me to engage with end users of the tools we build throughout the development process. For example, the Idaho Department of Fish and Game uses our computer-vision models for camera traps to help make informed decisions about the number of hunting permits to release every year in order to maintain sustainable populations. They're primarily interested in common, large species such as deer, elk, and wolves. As researchers who developed the model, we know that our model is good at detecting those types of larger mammals and, when used with a high confidence threshold, the rate of missed large mammals is very low. For them, because they capture millions of images a year and the risk of missing a small percentage of the animals is slight compared to the cost of reviewing every image manually, they only consider animal detections with high confidence. The Nature Conservancy (TNC) is using the exact same model to detect invasive rodents on islands, including Santa Cruz Island off the coast of California. A single rodent can lead very quickly to many rodents, which can rapidly devastate island bird populations. In this case, a missed detection could have serious consequences and the animals themselves are small, making them more challenging to detect. TNC therefore uses our models with much lower confidence thresholds and invests more human time in verifying the results and removing false detections that occur at lower confidence values. It's important to understand the end-use cases and these tradeoffs between risk and human effort (which is expensive) to communicate to stakeholders how they might use computer vision models effectively and safely in their workflows.
Data collected for ecology, environmental science, and climate, pose novel, complex challenges for the computer vision community. However, computer scientists working with these data in the absence of valuable knowledge from those scientific communities can lead to the development of methods that miss the mark and ignore the complex risks of deployment of automated systems in the real world. We need to build tools together with stakeholders and scientists across disciplines to enable us to effectively and reliably tackle the large environmental challenges we face, such as biodiversity loss and climate change. This interdisciplinary approach can enable us to ask completely new scientific questions about the world around us and the complex and fragile ecosystems we live in.
You can submit your own questions to the Caltech Science Exchange.