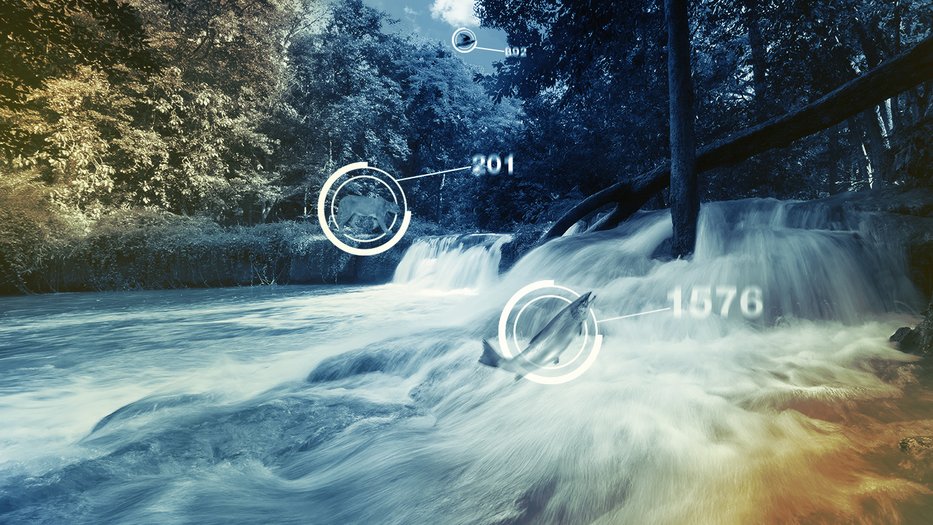
Ask a Caltech Expert: Machine Learning for Conservation
As part of Conversations on Artificial Intelligence, a webinar series hosted by the Caltech Science Exchange, two artificial intelligence (AI) researchers—Pietro Perona and Suzanne Stathatos—discussed AI’s potential as a powerful tool for wildlife conservation and biodiversity research.
Perona is the Allen E. Puckett Professor of Electrical Engineering at Caltech, and Stathatos is a graduate student who was a software engineer at Amazon and JPL, which Caltech manages for NASA, before coming to Caltech.
In conversation with Caltech science writer Robert Perkins, the engineers describe AI applications for identifying and tracking wildlife that offer fresh insights to biologists and other individuals interested in the environment.
Highlights from the conversation are below.
The questions and answers below have been edited for clarity and length.
What is computer vision, and how is it used?
Pietro Perona: When we open our eyes in the morning, we start seeing the world and understanding what is around us. And that's what we try to reproduce with computer vision. We want to give machines the same ability that we have to know about the world just by looking.
One thing we use our vision for is to understand the geometry of the world around us, for example, so we don't walk into obstacles. Another thing we use it for is recognition, so that we can categorize objects in the world and know how to interact with those objects. And, of course, we use it for social interactions. For example, if I look at you, Robert, I see that you're paying attention to what I'm saying, and you're not too confused.
We would like to give machines the ability to see so that they can reproduce all of these abilities that we have and better interact with people.
Why is it hard for computers to identify an object just by looking at it?
Pietro Perona: When we observe something with our eyes, it generates an image that is a representation of an object that may be very different from what is actually there. The image is produced by light that enters our eyes after having bounced off surfaces in the environment, and it carries with it the information of what it touched before entering our eyes. This representation is based on our perspective and on the lighting that is present. There is a lot of work to be done to decode what the image is telling us. And it turns out that more than half of our brain is dedicated to vision. We don't realize it, but our brain is working on vision more than it's working on almost anything else—language or proving theorems or whatever else we do during the day.
Suzanne Stathatos: Also, humans are constantly evolving and changing models. With age and with time, we learn a lot of new sensory inputs. If you have to train a computer model in computer vision, it doesn't have years and years and years of examples to work from. And we're trying to train computer vision models to learn about the world but with far fewer images than the human brain has collected.
What are some ways that AI can help with ecology and conservation?
Suzanne Stathatos: I've worked on a project that is monitoring salmon populations in the Pacific Northwest using sonar imagery. Fisheries throughout Alaska, Washington, California, and Oregon have already started putting sonar cameras in riverbeds to track salmon as they swim from their spawning sites, for both ecological and economic reasons. They watch how many fish swim across the video frame at a time and then count those as they swim by. We're trying to automate that process so that fisheries' resources can be alleviated, and they can work on other things while we count the fish that swim upstream. It's challenging, because the fish themselves aren't necessarily individually identifiable and their swim pattern is roughly the same. So unlike detecting [something] like a pedestrian versus a car, which are two distinct objects against very distinct backgrounds, these are much more difficult to detect and then track.
I've also worked with a student who wanted to understand how walrus populations are responding to the changing Arctic. Using remote satellite imagery and a computer vision approach to count brown pixels [the walruses] against the white background, we were able to start getting the baseline of the walrus population, which is critical to understanding how the population is shifting.
It sounds like it's not just about saving grad students' labor, it's also about getting at data we wouldn't otherwise have.
Suzanne Stathatos: Exactly. It's saving grad students' labor but also letting them answer questions that they might not otherwise be able to answer. They can approach the data in a different way and maybe address questions about things that they don't know that they don't know.
Pietro Perona: Let me tell you about something else, which is iNaturalist. So, iNaturalist is an app that anyone can download to their smart device, and typically people download it to their smartphone. The app was developed by Scott Loarie at the California Academy of Science to connect naturalists and amateur field biologists, and allow them to help each other identify plants and animals. We have added to iNaturalist the ability to interpret images automatically so that anyone can now identify plants and animals. This is mainly work by Grant van Horn, who was a student in the lab.
The idea was that when we take hikes and we are out in nature and see a plant or an animal, we would like to know which species is it. Is it a rare species? Should it be here at all? And so on. Using your phone, you can grab a picture of this animal or plant and have your phone classify it for you and suggest a number of species that it could be. And then amongst these, you can choose the one that is most likely. It's a social network, so you can post your observation, and other people who are interested in that species or that location can come in and look at your observations and can contribute their thoughts on which species is it, and correct your determination of the species or the machine's determination of the species. Behind the scenes, we've built a statistical machinery that associates with each person a description of their knowledge and an estimate of how often are they right when they spit out a species identification in a certain domain.
As this technology improves, we should also think about potential ethical and privacy concerns, especially with things like facial recognition. Have you run into any tricky issues?
Pietro Perona: It's something that we think about. Face recognition, whether you like it or not, is as successful as the applications that we were talking about. A system to recognize faces is much more accurate than any human and could be a wonderful tool. For example, in the U.S., there have been reports by the National Academy of Science on how often eyewitnesses in a crime make the wrong identification and people end up in jail due to racial prejudice, for example. It's really good to have something that is better than humans and something that you can test for things like prejudice or bias. You can know if the system is biased or not, and, if it is, you can fix it. The hope is to have systems that help us have a more just society, one that works better. But it's true that they can be misused.
Suzanne Stathatos: An interesting application that's particular to conservation is related to camera traps. [Camera traps are motion-triggered static cameras that are placed in the wilderness to monitor wildlife.] There's this question of should something be done if a camera trap sees a poacher, or should it recognize a poacher? And then there's a flip side. Is this the responsibility of the computer vision researcher? And then also things like geo locations, especially when you're dealing with species that are going extinct. You don't want to publish their exact GPS coordinates because if this data is being released, then opportunists can take it and say, "Oh, I can find a rhino at this exact location." So we have to make sure that aspects of the data are not accessible.
Here are some of the other questions addressed in the video linked above:
- What first interested the researchers in the field of applying computer vision technology to ecology problems?
- How do I get started in the field of computer vision? (Includes information about joining the AI for Conservation Slack community.)
- What other potential applications exist for this technology?
- And what unrelated and exciting applications also exist for computer vision?
Learn more about artificial intelligence on the Caltech Science Exchange.