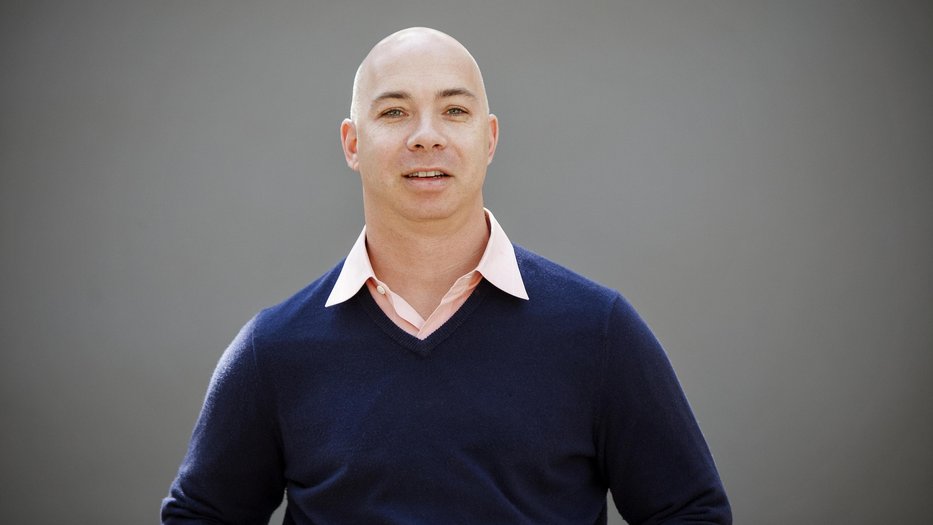
Ask a Caltech Expert: Jonathan Katz on Gerrymandering, Election Prediction & Counting Ballots
Is there a way to measure the fairness of district boundaries to prevent gerrymandering?
Among political scientists who study elections, the standard for measuring the partisan fairness of district boundaries is called partisan symmetry. The concept is fairly easy to define in the abstract. Suppose it's the case that when Democrats win 55 percent of the vote, they get 65 percent of the seats. We'll call that fair as long as, if the situation were reversed and Republicans won 55 percent of the vote, they too would get 65 percent of the seats. Note that this is not proportionality. If you had a truly proportional election system, each party's share of seats would equal that party's share of votes. However, district systems such as what we have in the United States or in the United Kingdom or Canada tend to be antiproportional. That is, the party that gets a plurality of votes tends to get a bonus—they get extra seats. That's fine if the bonus is symmetrical.
So, the easy part is how to define fairness. The hard part is knowing whether a given map is fair or not. Usually, you have to do some statistics in which you project a counterfactual, or hypothetical, outcome based on a statistical model.
One approach, which seems naïve on the front end but works surprisingly well, is uniform partisan swing. Suppose we observe an election in which the average Democratic vote share was 55 percent. We'd like to know how many seats the Republicans would win if instead they got 55 percent of the vote. Would the result be symmetrical?
Assuming there are only two parties, we know that if Republicans got 55 percent of the vote overall, Democrats would get 45 percent of the vote, or 10 percentage points less than in the observed election. Uniform partisan swing says we can take election results across districts and adjust them up or down by the same 10 percentage points, and that's what we would have observed had this hypothetical election happened.
To complicate the process further, it's important to remember that partisan fairness is not the only objective we care about. We also want to make sure maps appear reasonable in an aesthetic sense. We might also care about the competitiveness of elections and about maintaining communities of interest. Even when we try to establish procedures to make redistricting more fair, there's a lot at stake, and so there are incentives for political actors to try to game the rules. But partisan fairness can definitely be measured. Political scientists do this routinely.
What makes presidential elections so difficult to accurately forecast?
As a statistician or researcher conducting a survey of a population, I want to define that population and then take a representative sample. When working with populations for which there's a census, for example U.S. households, that's easy. In contrast, a fundamental problem with election polling is defining the population. Not everyone votes, and turnout fluctuates between election cycles. There's no census, or defined population, of voters to sample from.
Pollsters have different strategies for getting around this problem. For example, in most states you can get a list of registered voters, and some polls will sample from that list in what are called registered voter samples. The problem here is that not all registered voters vote. So, to get a representative sample, I'm going to have to create a statistical model to help predict how likely it is that a given individual will, in fact, vote. The challenge is that reasonable statisticians can have different views on what a reasonable voter model is.
That's problem one: we can't define the population that we want to sample from. We're going to have to make some assumptions, and different pollsters make different assumptions.
The second problem in forecasting presidential elections is that a poll represents a snapshot of public opinion today, but what we really care about is what happens on the first Tuesday after the first Monday in November. Things change quickly, even in a relatively brief space of time, and, clearly, the farther out we are from Election Day, the more uncertainty we have to accept.
The third problem is, in general, that response rates to surveys are way down. It used to be, back when Gallup was the only business in town, people were more willing to participate in surveys. Today you might see low-quality polls with a 3 percent or 4 percent response rate. Now you, as a researcher, have to make heroic assumptions about the people you could get on the phone versus the people you couldn't. There are statistical fixes, but, again, those fixes add another layer of assumption about which reasonable people might disagree. And it turns out, how you disagree can have profound impacts on your polling results.
Why is it so hard to count every ballot?
The voting process is actually very complicated, which most people don't think about. Were you on the voting rolls? Did you get to vote? Did you have to cast a provisional ballot? If you cast a provisional ballet, was it counted? If your ballot was counted, was your intent accurately recorded? These are all factors that come into play.
Manual counts, for example, may seem relatively simple because counting is easy. However, what we know from studies of human behavior is that humans are really bad at repetitive boring tasks. People just make mistakes. When elections are closer than one-half or one-third of a percentage point, that's basically a tie. Furthermore, small rules about which ballots are counted as valid can also have an impact.
Even when a count is completely computerized, there can be problems. There could be a bug in the code. Or, a vote that is recorded by a local machine might not be transmitted to the central server to be tabulated.
There is also the question of measurement resolution, which scientists talk about all the time. Whenever you take a measurement, you consider how accurate that measurement is: How accurately can I titrate a solution? How accurately can I weigh a sample? Every instrument in a laboratory has a precision level. So do voting machines, so does the voting process itself. Because we're dealing with machines, we tend to think there's no uncertainty, but that isn't the case.
—Jonathan N. Katz, Kay Sugahara Professor of Social Sciences and Statistics
You can submit your own questions to the Caltech Science Exchange.